AI seems to be the talk of the town, in every town! Can it write better than we can? Can it create art? Will it take our jobs?
In some cases, it may seem like AI is just like a human and can solve problems even faster than humans. But what happens if you ask it some basic questions: what time is it? What is the product of 12345 x 6789? It will fail in both cases. Not so smart, right?
Let’s explore what AI is now vs. one direction it might be headed (stress on the “might”): from single-step problems to multi-step problems.
Single-step problems
Thus far, the AI that we have experienced is solving problems that are single step in nature. The AI model takes some input, computes based on that input, and provides an output. For example, the input could be a sentence or a paragraph, and the output could be whether the sentiment of the sentence was positive or negative. Or, the input could be data on loan defaults for the last ten years, and the AI will output patterns in the data indicating which borrowers are more likely to repay their loans.
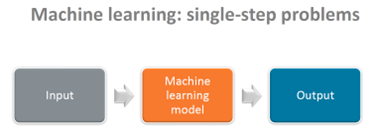
But there are many things in the world that can’t be solved in a single step. AI is moving into the realm of multi-step problems.
Multi-step problems
In the telling time example, humans don’t know what time it is from our memories. We consult our watch or phone to know what time it is. And likewise if you ask us to multiply large numbers together, we don’t know the product from our memories—we use a calculator. Computers should eventually be able to do the same thing—consult an outside tool to complete the task. That is what could be next for AI—computers can learn to interact with the world, drawing conclusions and making predictions accordingly. This possible future for AI is called action-oriented AI or autonomous AI.
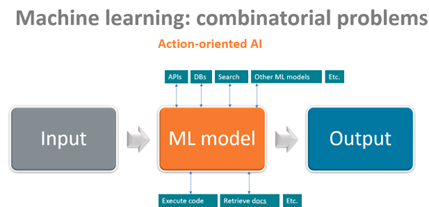
For example, if AI is helping an analyst perform research, the AI can start with what it knows from “memory.” This would be a single-step problem: it would draw from its training data to produce an output. But then, for more robust output, the AI might include information from some internet searches, pull in current information from an API, etc. Those multiple steps to create an output is where AI could be going next.
White paper | Considering AI solutions for your business? Ask the right questions.
Remember that predictions, including my own, are inherently uncertain. They are based on our observations and speculations about how the future might unfold. While the techniques in the field of AI are still evolving and far from being mature, we are witnessing numerous advancements. This area is progressing rapidly, and if it develops as anticipated, it has the potential to address currently insurmountable challenges. It may redefine what is achievable and mark the beginning of an exciting new era in the realm of AI.
Note: Other potentially noteworthy advances
Additionally, there is likely to be progress in various other areas of AI, including:
- Foundation models: Expect improvements in foundation models across all modalities, including multi-modalities and reinforcement learning.
- Zero-shot capabilities: Anticipate enhancements in the ability of foundation models to perform zero-shot learning, enabling them to generalize to unseen tasks or domains.
- Sample efficiency: Efforts will be made to enhance the sample efficiency of foundation models, enabling them to achieve similar performance with fewer training examples.
- Reliability and control: Ongoing research aims to enhance the reliability, control, and deeper reasoning capabilities of AI systems.
- Hardware: Expect the development of better and more affordable hardware dedicated to training and inference, further enabling advancements in AI.
- Model architecture and optimization: The architecture of AI models will improve, along with better optimizers, allowing for larger sequence lengths and better performance.
The opinions provided are those of the author and not necessarily those of Fidelity Investments or its affiliates. Fidelity does not assume any duty to update any of the information.
1093488.1.0