The field of artificial intelligence (AI) has come a long way since its inception, evolving from simple rule-based systems to complex deep learning models capable of learning patterns from vast amounts of data. This blog delves into the progression of AI over the decades, highlighting the shift from handcrafting features and predictions to the current era of pre-training, fine-tuning, and the eventual move towards autonomous and generative AI. Notable milestones such as AlexNet, AlphaGo, DALL-E, StableDiffusion, PaLM, GPT-3, and GPT-4 demonstrate the remarkable advancements in AI capabilities.
Handcrafting features and predictions
In the early days of AI, researchers and engineers would handcraft features and predictions, meaning they would manually design algorithms that extracted relevant information from the input data. These algorithms were largely based on human expertise and intuition and were tailored to specific tasks, such as image recognition or natural language processing.
However, these handcrafted algorithms faced limitations, including the “curse of dimensionality” and difficulties in scaling up to handle more complex tasks. They were often brittle, difficult to generalize to new tasks, and required significant human effort to design and maintain.
Emergence of deep learning and neural networks
The limitations of handcrafted features led to the development of deep learning models, which are capable of automatically learning features and predictions from raw data. These models, based on artificial neural networks, consist of layers of interconnected neurons that process data using activation functions and learn through a process called backpropagation. Deep learning models have been hugely successful in a wide range of applications, such as computer vision, speech recognition, natural language processing, and even game playing.
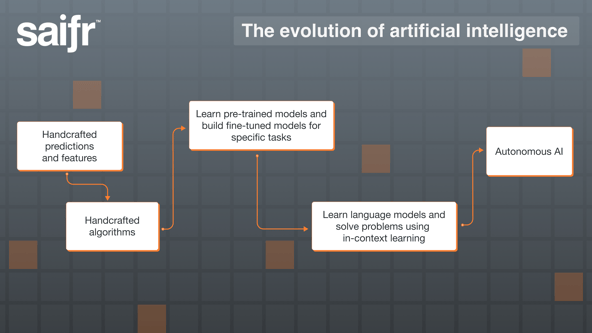
Pre-training and fine-tuning
Despite the success of deep learning models, they require a large amount of labeled data to achieve high performance, which can be both time-consuming and expensive to acquire. To address this challenge, the AI community has developed methods such as pre-training and fine-tuning.
Pre-training involves training a neural network on a large dataset, often unsupervised or with minimal supervision, to learn general features and representations. The pre-trained model can then be fine-tuned on a smaller, task-specific dataset to achieve better performance on the target task. This approach reduces the need for large amounts of labeled data and enables the transfer of knowledge from one domain to another.
Generative AI: large language models (foundation models)
A major breakthrough in AI research has been the development of generative models, such as the state-of-the-art large language models (LLMs) GPT-3 and its successors, and image synthesis models like DALL-E, StableDiffusion, etc. These models have shown remarkable capabilities in generating human-like text, images, and other creative works.
White paper | Considering AI solutions for your business? Ask the right questions.
GPT-4, developed by OpenAI, is a powerful LLM that can perform a wide variety of tasks, from generating text to answering questions and even programming. Its massive scale and pre-training on diverse data enable it to generate contextually relevant and coherent responses, making it a significant milestone in AI research.
DALL-E, also developed by OpenAI, is a diffusion-based model that can create realistic or artistic images from textual descriptions. This innovative model demonstrates the potential of AI in creative fields and opens up new possibilities for artistic expression and design.
Towards autonomous AI and challenges
The advancement of AI research and applications is moving towards the direction of autonomous AI, which aims to create systems that can learn and adapt on their own without much need for human intervention. Autonomous AI systems will likely be capable of continuous learning, self-improvement, and decision-making in complex and dynamic environments and have the ability to solve combinatorial problems.
Key components of autonomous AI include reinforcement learning, reinforced learning from human feedback (RLHF), self-supervised learning, generative AI, and active learning. Reinforcement learning allows AI systems to learn from trial and error, while unsupervised learning enables them to discover patterns in data without explicit labels. Active learning, on the other hand, allows AI systems to intelligently select the most informative samples for learning, reducing the amount of data needed for training.
As AI continues to advance, potential challenges and ethical concerns may arise, including issues related to privacy, fairness, accountability, and the potential loss of human jobs. Addressing these challenges will be crucial in ensuring the responsible development and deployment of autonomous AI systems.
Conclusion
The field of AI has progressed significantly over the decades, transitioning from handcrafting features and predictions to leveraging deep learning models that learn from data. As AI research continues to advance, we are moving towards a new era of pre-training, fine-tuning, in-context learning, and eventually autonomous and generative AI systems that can learn, adapt, and make decisions on their own when they are given a goal to accomplish. These developments hold great promise for future applications, but also raise important challenges and ethical considerations that must be addressed to ensure responsible and beneficial AI innovation.
Are you considering AI solutions for your business? This white paper outlines essential questions to ask when evaluating AI vendors.
The information regarding ChatGPT and other AI tools provided herein is for informational purposes only and is not intended to constitute a recommendation, development, security assessment advice of any kind.
The opinions provided are those of the author and not necessarily those of Fidelity Investments or its affiliates. Fidelity does not assume any duty to update any of the information. Fidelity and any other third parties are independent entities and not affiliated. Mentioning them does not suggest a recommendation or endorsement by Fidelity.
1085488.1.0